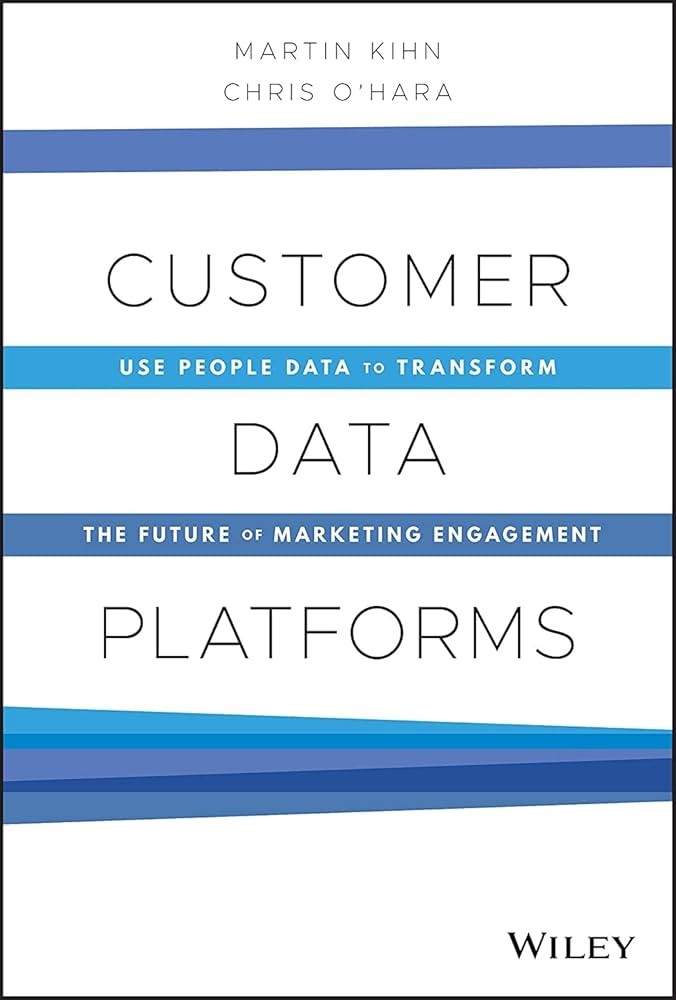
Review
Marketing is more about data and technology than ever. MarTech helps companies get the right customer, the right message at the right time. Customer Data Platforms (CDPs) occupy the center of the modern marketing tech stack. CDPs promise to solve some of marketing’s biggest problems.
If you understand CDPs and the systems they interact with then you understand digital marketing. This book is incredibly useful if you’re new to (or a little rusty on) the world of customer data management.
Key Takeaways
The 20% that gave me 80% of the value.
- In attempt to respond to changes in technology companies quickly added new teams and new tools to their marketing operations. That in turn caused:
- ‘Martech Frankenstack’
- Data Silos
- Fragmented teams: mobile, brand, email
- Difficulty coordinating the customer experience
- The ideal marketing infrastructure is easy to define but hard to achieve:
- A unified user profile
- Smart segments – perform segmentation, machine learning
- Plan and react capability – campaign management and decision capability
- Engagement – directly engage with the customer (email, SMS)
- Optimization – capturing signals, recommending improvements, reporting results
- Without effort and strategy → data becomes siloed:
CRM, Loyalty Service | Salesforce, AWS, SQL Server |
Email, Mobile, Social | Salesforce – Marketing Cloud, LinkedIn, Instagram, YouTube, Facebook |
Sales | Salesforce – Commerce Cloud, Magento, IRI, SAP HANA, Nielsen |
Web Analytics | Adobe Analytics, Google Analytics |
Paid Digital Ads | Instagram, twitter, snap, Google Ads, Google AdSense, Amazon |
Programmatic Ads | Turn, Moat, The trade desk, TubeMogul, AppNexus, |
Customer Databases | Data lakes, propensity scores, internal models |
Agency Reporting | Excel, google sheets, PDFs. |
- To make the most of your data → you need to break silos → by resolving customer data + creating data portability across applications. Break down the people silos that prevent collaboration by unifying the data completely.
Enter the Customer Data Platforms
Main functionalities:
- store customer data and do analytics
- enable use of that data (real-time engagement system)
How CDP compares to other products:
Data Warehouse | Custom Integration | DMP | CRM | CDP | |
Unified Customer Data | ✅ | ❌ | ❌ | 🔶 | ✅ |
Persistent | ✅ | ✅ | ❌ | ✅ | ✅ |
Packaged System | ❌ | ❌ | ✅ | ✅ | ✅ |
Real-time Capability | ❌ | 🔶 | ✅ | ❌ | ✅ |
Open Access | ✅ | ❌ | ✅ | 🔶 | ✅ |
The CDP is an evolution of the CRM category.
The Five CDP Capabilities:
- Data Collection
- Collect a variety of customer data
- Batch / API / SDKs / Tags
- Both Known and Unknown
- Interoperability is key to get to the ‘golden record’
- Data Management
- Classification and Schema
- The manipulation of disparate customer data into a common format
- Unified Profile
- Move from singular contacts – to rich profiles
- Aligns all data → including loyalty, call center, third party
- Unification and fidelity of the profile are really important
- Segmentation and Activation
- We need segments – because we can’t quite deliver a 121 personal experience yet
- The challenge of segmentation previously – has been the multiple places it happens
- Unifying where marketers do segmentation – making them available to others
- CDPs can then activate those segments – service as a source of truth for decision making
- Insights / AI
- Use AI to build segments
- Predict the right content, the best time of day to trigger a message, or the engagement score
- As CDPs are the source of truth – they are the source of data for AI
- Anonymous to Known → Start capturing data when the customer is still anonymous or pseudonymous
- A system of engagement → providing real-time engagement, such as channel optimization, next best-offer management, and dynamic creative optimization
- A system of insight → providing a more persistent single view of the customer or customer 360, for the purposes of in-depth analysis, modelling and measurement
- Ultimately all of this is in service of creating a more personalized customer experience.
- Marketing = Right Customer + Right Message + Right Time
- Typically, lack of data isn’t the issue. It’s data unification that’s the problem.
- Known Customer Resolution → taking data from a number of sources, and creating a single, unified profile for every customer (the Golden Record)
- Data Portability → Once you’ve resolved customer data – you want to make available to other systems
A Platform, not a Product
- To unify and integrate the landscape, using customer data as the glue
- To enable existing MarTech products → and enable a new generation
- The operating system that marketing and advertising plug into
Majority of CDPs
- System of insights (learning about the customer OVER interacting with them)
- Sorting out data silo problems
- Golden record of the customer
- Query data easily
- Adding propensity scores (receptiveness to marketing)
- Getting a better understanding of customer data – unified, connected, queryable
- Serving as the single source of customer truth – aggregation point for many different sources of data
- System of engagement (using data to influence the customer journey)
- Requires real-time customer profile store, ready to be accessed by other applications in milliseconds
- Fast-moving, near real-time. Managing real-time profile of customer data – activating them
- Requirements:
- Enterprise Holistic CDP – combining insights and engagement
- Known and unknown data unification – it’s not possible to truly understand customers without accounting for their interactions across PII and pseudonymous data modalities
- A business user friendly UI – unlocking the value of data to more people
- A platform ecosystem – take advantage of new applications
- Platforms spur innovation
- lower the barrier for entry for new entrants
- more specialty apps
- Platforms spur innovation
Consent is key
- Customers are increasingly resistant to data collection
- Customers are increasingly resistant to advertising
- 4 parties involved in data privacy: Web Browsers + standards bodies, government regulators, consumers and companies
The Seven Principles of GDPR
- Transparency → the consumer should know what data is being collected by whom
- Purpose limitation → data can only be used for the explicit purpose granted by the customer
- Data minimization → only as much (and no more) data can be collected to serve the purpose above
- Accuracy
- Storage limitation → limits to how long data can be retained
- Security → must be stored in a way that it cannot be accessed by unauthorized parties
- Accountability → the data ‘controller’ must provide ability to audit and edit data
6 ways to build trust
- Get consent
- Restrict processing of data
- Honor the right to be forgotten
- Allow subject requests for data stored
- Keep data secure
- Capture data use preferences
- A healthy majority of customers are willing to share data in return for relevance – as long as it is collected and used in a way that makes them feel comfortable
Transform your company using data
- Goal- get better at understanding and engaging with their customers:
- Know your customer
- Personalize every interaction
- Engage across channels and surfaces they touch
- Measure everything and use that data to improve
- Transformations come together around people, process and technology. While you need all three, the people are probably the most important aspect.
- You need a platform operating philosophy. A common language, enables creativity by allowing extensible model that lets people develop on them
- A platform approach is what gets value from the CDP
- Democratize data in the organization. Make data easily available to anyone who needs it
- Data unification for it’s own sake doesn’t provide value
- The idea is to produce a flywheel
- Tech gives access to highly enriched and unified customer data set
- Analytics team builds better customer segments
- Marketing activates segments
- Campaigns create more data that further enrich the dataset
- Better data leads to better segmentation. Better segmentation leads to better engagement. Better engagement leads to better data. Rinse and repeat
- A working maturity model
- Channel Coordination (single, multi, cross, omni)
- Engagement Maturity (segmented, lifecycle, individualized, connected)
- Segmented → by segment
- Lifecycle → by segment + funnel stage
- Individualized → customer + funnel stage + status
- Connected → profile + different systems + personalization
- From touch-points → journeys → experiences
- Journeys requires multi-channel and better identity capability and better lifecycle awareness
- Experience → move from channel based interactions to real time in the moment sending the right message and creative
Advertising
- You need to go beyond your own customers data to find and acquire new customers and fill the top of the funnel and drive new revenue
- Those customers are on social networks, they’re browsing your site anonymously
- How do you reach them? Through advertising – How do you target them? Using the DMP and building segments of pseudonymous consumers
- Why Pseudonymous data is still important
- The number of internet connected devices is only going to increase
- Most consumers interact with brands anonymously before they volunteer information
- Consumers digital exhaust signals can reveal a deep understanding of intent
- Ad networks let you send first party data to them – so you can target the customers you know (by email address) etc. Facebook will return a match about 70% of the time.
- CDPs therefore can define a segment and send it to facebook or google and execute an ad campaign against it
Beyond Marketing
- Customer data can make a better experience across marketing, support and sales
- An experience can either be seamless or disjointed, depending on how good companies are at using customer data
- Abandoned baskets are noticed and customers are emailed.
- Purchases are noticed and customers are emailed (new purchase flow)
- Start with suppressing adverts to customers who’ve already purchased that product to reduce costs
- Once a customer has purchased we have high value deterministic data to develop propensity models
- Connect this data to unknown users. You could send emails for abandoned baskets
- Combining highly granular attributes of unknown visitors with known customer purchase data can yield effective predictive models
- The purchase isn’t the end of the journey → but the seed that can grow into a new top-of-funnel campaign
- The single source of truth → creates a platform that others can build on
- House-holding → target households not just individuals
- Track targetable attributes → engagement of emails can help with propensity modelling
- How can marketing data be an input into the sales, service and commerce functions
- Every team gets more in value than they individually contribute (Platform / Center of excellence mindset)
Other Highlights
- Customer data in a warehouse serves no purpose – it must be activated to yield value
- Top Uses for AI among marketers
- Personalize individual channel experiences 80%
- Improve customer segmentation / lookalike audience modelling 77%
- Automate interactions over social channels / messaging apps 76%
- Drive next best actions in real time 76%
- Surface insights from data 78%
- Clever targeting: Inventory availability – so you have the stock to follow through
In-depth Summary
Introduction
The marketing space is getting harder
- Loyalty to consumer brands is declining
- Cost of advertising is rising
- People are becoming less receptive to adverts (40% don’t/or barely respond)
The Impact of Personalization
- The Personalization Flywheel:
- Personalization → better experience → more usage → more data → better personalization
- Amazon’s recommendations are 2x better than other large retailers – because of data!
- Consumer expectations
- Consumers want more relevant messaging and promotions
- Consumers expect personalization
- Consumers (69%) expect connected experiences (entering information once)
- Personalization works
- Example: pizza shop increases conversion by 16% after using preferences in emails
- Better personalization on e-commerce → +15-20% conversion, +30% engagement
- Targeting ads based on website visits → +200% click through
- Personalized emails → +6% open rate
- From segments to personalized → +20% lifetime value, +30% engagement
Challenges of Personalization
- Privacy paradox: customers want personalized experiences → but are wary of providing access to the data that enables them (behavioral, attitudinal, demographic)
- Privacy and regulatory challenges
- Consumer attitudes to data collection, storage and access are changing
- A decline in consumer trust and increase in ad-avoidant and marketing-hostile behavior
- GDPR in Europe and CCPA in California
- People are OK with the right data being shared with the right people for the right reasons
- Marketers are becoming more mindful with personalization
- Managing and connecting data
- If we had a magic data wand → personalization would be unlocked
- Disconnected data is a key challenge
- Companies are unhappy with their data:
Data Quality | 37% |
Data Timeliness | 34% |
Data Integration | 34% |
Consent Management | 34% |
Identity Reconciliation | 33% |
Chapter 1 – Customer Data Platforms
CRMs have been improving: response rates, satisfaction, throughput, sales, market share, closing rates → since the 90s. They upped the game for many consumer companies. CDP is an evolution of the CRM category.
Customer Data Platform must provide:
- Anonymous to Known
- Start capturing data when the customer is still anonymous or pseudonymous
- Includes capabilities associated with DMP (Data Management Platforms)
- Insights and engagement
- A system of engagement → providing real-time engagement, such as channel optimization, next best-offer management, and dynamic creative optimization
- System of insight → providing a more persistent single view of the customer or customer 360, for the purposes of in-depth analysis, modelling and measurement
- For personalized, trusted, one-to-one messaging and marketing, yielding a better customer experiences.
- Reliable, future-proof and customer driven
Chapter 2 – The Customer Data Conundrum
Marketing = Right Customer + Right Message + Right Time
Typically, lack of data isn’t the issue. It’s data unification that’s the problem.
Without effort and strategy → data becomes siloed
Where is data stored? Who can use it? For what can it be used?
CRM, Loyalty Service | Salesforce, AWS, SQL Server |
Email, Mobile, Social | Salesforce – Marketing Cloud, LinkedIn, Instagram, YouTube, Facebook |
Sales | Salesforce – Commerce Cloud, Magento, IRI, SAP HANA, Nielsen |
Web Analytics | Adobe Analytics, Google Analytics |
Paid Digital Ads | Instagram, twitter, snap, Google Ads, Google AdSense, Amazon |
Programmatic Ads | Turn, Moat, The trade desk, TubeMogul, AppNexus, |
Customer Databases | Data lakes, propensity scores, internal models |
Agency Reporting | Excel, google sheets, PDFs. |
Known data = PII = Personally identifiable information
- Valuable – typically stored in a CRM
- Often stored in a CRM, often fragmented (sales support, marketing, commerce) and rarely combined stored in a way that can be made us of in a customer journey.
Customer Relationship Management (CRM)
- is the OS for customer data that the sale organisation plugs into
- should be the source of truth
To make the most of your data → you need to break silos → by resolving customer data + creating data portability across applications
Known Customer Resolution → taking data from a number of sources, and creating a single, unified profile for every customer (the Golden Record). FirstName and First_Name need to be reconciled. You need to create a data model to standardize those fields – and map them accurately
Data Portability. Once you’ve resolved customer data – you want to make available to other systems. Deciding what data to port – to what granularity is an art. The CDP aims to solve unification of customer data – and provisioning a golden record that marketers can rely on an leverage.
Unknown data
- Prior to logging in – or giving PII – it’s hard to know who’s doing what, customers have many devices, customer journeys are often multi-device
- Data Management Platforms (DMPs) were created to solve identity. Resolving unknown customer data is much harder
- GDPR and other privacy regulations also make this is a difficult area
Fact Check:
- 4.3 connected devices per US adult
- 41% to 67% of online purchases are the culmination of a multi-device journey
- 66% retailers can’t tell a customer has switched devices during a path to purchase
- Trends → more unknown data, more multi-device usage
Marketers might no longer be buying DMPs – but they’re still going to want and need to capture signals from the beginning of the buying journey
Cross-Device Identity Management → recognizing customers across devices, gather contextual and behavioral data about the customer → add to a segment:
- You can get a rich view of the customer doing this
- You need a mechanism for tracking consent and the right to be forgotten
- You need to capture different consent flags – else you create a binary system, 100% in or out
Types of Consent: Data collection, analytics, targeting, cross-device, data sharing, re-identification
Connecting the known and unknown – the identity problem. Connecting them gives you a full view of the customer funnel.
Build awareness and interest (top of funnel) → desire and action (at bottom of funnel)
The CDP can connect known and unknown customer data:
- Direct Engagement and Known (CRM) : Direct Mail, Email, Sales, Call Center, Commerce, SMS
- Passive Engagement and Anonymous DMP: Display, Video, Mobile Web, Mobile App, SSP/DOC, Social
Data Onboarding → the process of matching unknown (cookie / device ID) to known (person/ email):
- LiveRamp specialized in just that
- Company provides email addresses (100m) and gets cookies in return (40m), match rates are typically 30-90%
- Without the connection – brands suffer from observational bias – only seeing what they can see
People Silos – organization structures can often dictate the data silos.
Break down the people silos that prevent collaboration by unifying the data completely -> then build incentives for everyone to enrich the golden record -> having the data in one place will encourage customer-driven thinking and collaboration
The History of Customer Data
History of Marketing (1870 to present)
- 1870 → Direct mail circulars
- 1894 → Sears catalog
- 1958 → Lester Wunderman coined ‘Direct Marketing’
- 1961 → ‘Society of the Divine Savior Data Processing Center’
- Crude predictive models and LTV calculations were made using IBM processors and tape
- 1970 → E.F.Codd invents the relational data model
- 1980s → Database Marketing
- and into the 90s → Relational databases and SQL enabled personalized marketing.
- 90s – 00s → Digital Marketing Wave 1: the rise of the internet and email
- 1990s → Marketing Automation
- 00s – 10s → Digital Marketing Wave 2: the rise of mobile and social
- 2000s → Multichannel Campaign Management
- 2010s → CDP
The timeless elements of the marketing stack:
- Data input – ingest data from multiple sources
- Storage – persistent available storage
- Data processing – transform data into useful forms
- Activation – get signals from the data
- Engagement – send them to different channels
- Measurement – track and optimize results
Customer Relationship Management (CRMs) emerged to automate the process:
- No need to write SQL
- Easy campaign management
- Automated engagement (sending of emails)
- Expanded to data beyond email creating a feedback loop (A/B testing)
Customer Engagements Channels → Email / Direct Mail / SMS. Email is still popular → it’s multichannel and easily measurable
- To keep up companies quickly added tools and teams. That lead to:
- ‘Martech Frankenstack’
- Data silos
- Fragmented teams: mobile, brand, email
- Difficulty coordinating the customer experience
- The need for Customer Data Platforms was clear:
- to store customer data and do analytics
- to enable use of that data (real-time engagement system)
- Ideal marketing infrastructure would include:
- A unified user profile
- Smart segments – perform segmentation, machine learning
- Plan and react capability – campaign management and decision capability
- Engagement – directly engage with the customer (email, SMS)
- Optimization – capturing signals, recommending improvements, reporting results
Ideal Marketing Architecture:
Engage Across The Entire Customer Journey | Ads · Social · Email · Mobile · Website · APIs |
Personalize the Engagement | + Plan Campaign Management + React Real-Time Interaction Management |
Know the person | Smart Segments → Segment Discovery, Cont. Recommendations |
Analyze the results | Unified User Profile → Identity Service · Metadata Management |
Chapter 3 – What is a CDP, Anyway?
- 2013 the term CDP was first coined by David Raab
- 2016 the first CDPs became available on the market
- 2019 CDPs started to become popular
- Suffered from confused definitions, blurred boundaries and vendor hype
- Key Components of a CDP:
- Acquire – natively collect and extract data from many source s
- Process – cleanse and manage attributes and IDs
- Expose – make data available in a persistent store
- Analytics and decisions – execute decisions and perform predictive analysis
- Delivery – send signals to engagement systems
- This is nearly all of marketing, so it’s ambitious.
- CDPs are there to organize customer data and make it available to the systems that need it.
- Promises made by the CRM vendors of the past – are similar to those made by CDP vendors today
- CDPs aren’t new → they’re the latest evolution of CRM and expanding beyond just marketing.
- What marketers wanted a CDP to do:
- Cross-channel campaign management (frequency and sequence of messages)
- Segmentation (better segments, LTV segmentation, High Value Customer identification)
- Loyalty (offer management and loyalty)
- Measurement (ROI of marketing tactics)
- Features they expected:
- Profile building – known or unknown
- Data ingestion – from common systems
- Identity management – tie records to existing accounts, fuzzy matching, cleansing, de-duplication, household mapping
- Normalization – manual and automated for attributes in tables
- Marketer friendly UI – SQL not needed, drag and drop segmentation / rule building
A PLATFORM NOT A PRODUCT
- To unify and integrate the landscape, using customer data as the glue
- To enable existing MarTech products → and enable a new generation
- The operating system that marketing and advertising plug into
Five CDP Capabilities
Data Collection | Collect a variety of customer data Batch/ API / SDKs / Tags Both Known and Unknown Interoperability is key to get to the ‘golden record’ |
Data Management | Classification and schema The manipulation of disparate customer data into a common format |
Unified Profile | Move from singular contacts – to rich profiles Aligns all data → including loyalty, call center, third party Unification and fidelity of the profile are really important |
Segmentation and Activation | We need segments – because we can’t quite deliver a 121 personal experience yet The challenge of segmentation previously – has been the multiple places it happens Unifying where marketers do segmentation – making them available to others CDPs can then activate those segments – service as a source of truth for decision making |
Insights / AI | Use AI to build segments Predict the right content, the best time of day to trigger a message, or the engagement score As CDPs are the source of truth – they are the source of data for AI |
Majority of CDPs
- System of insights (learning about the customer OVER interacting with them)
- Sorting out data silo problems
- Golden record of the customer
- Query data easily
- Adding propensity scores (receptiveness to marketing)
- Getting a better understanding of customer data – unified, connected, queryable
- Serving as the single source of customer truth – aggregation point for many different sources of data
- System of engagement (using data to influence the customer journey)
- Requires real-time customer profile store, ready to be accessed by other applications in milliseconds
- Fast-moving, near real-time. Managing real-time profile of customer data – activating them
- Enterprise Holistic CDP – combining insights and engagement
- Known and unknown data unification – it’s not possible to truly understand customers without accounting for their interactions across PII and pseudonymous data modalities
- A business user friendly UI – unlocking the value of data to more people
- A platform ecosystem – take advantage of new applications
- Platforms spur innovation
- lower the barrier for entry for new entrants
- more specialty apps
- Platforms spur innovation
- This new platform is going to create a second golden age or MarTech. Anyone is going to be able to spin up specialty apps that leverage CDPs
Chapter 4 – Organizing Customer Data
High level key steps for data management:
1) Data ingestion
- Identify the different sources of customer information across your systems
- Collect relevant data and point it towards a centralised architecture
- Batch processing: Asynchronous ingestion at bounded intervals. Good for high volume of data for specific time periods (e.g. daily point of sale purchase data)
- Stream processing: Synchronous ingesting of data stream (e.g. web analytics)
- Just because data is collected – doesn’t mean that it’s available. Often harmonization is required before it can be useful.
- 3 ways data can be ingested for use: Extracted, collected in real time, collected in batches.
2) Data Harmonization
- Ingested data requires transformation before it can be used for analytics, decisions or processes.
- Prepared, cleaned, modified, enriched so it can be fit for a business use case
- You need an agile, flexible harmonization strategy
- Harmonization is the process of mapping fields from different data sources that represent the same type of data
- You can either integrate by using middle ware or making changes at the source
- CDPs allow you to map data fields from different sources in a drag and drop way
- Best practice is to start with a standard data model – and customize it for your requirements. Doing so saves time and reduces errors.
- Salesforce uses CIM (Cloud information model), a subset of which is the customer information model
3) Identity Management
- Identity management seeks to reconcile customer IDs → using identifiers such as names and addresses, mobile phone numbers, emails, anonymous browser cookies etc
- Helps power more effective multi-channel engagement, improve targeting and creative tactics and makes measurement more accurate.
- Identity management can also help provide a more consistent experience for customers
- Marketers want to link devices and browsers to individuals because so much of the customer journey can happen on another device or before logging in.
- Acxiom, Experian, Epsilon and KBM maintain authenticated identities for almost every customer and business in developed countries
- Benefits of identity management
- Improved media effectiveness → Efficiency, targeting and audience extension
- More relevant content → Personalization of messages and offers, and sequencing across channels and devices
- Better analytical insights → higher profile accuracy for segmentation, predictive models, recommendations and measurement.
- More informed governance → the ability to meet consumer and regulatory requirements for opt-outs, preference management etc.
- The Spectrum of Identity
- Identity isn’t about device matching and it isn’t as easy as classifying at known or unknown.
- Pseudonymous identifiers: point to locations and devices not a person (IP address, Device ID)
- Known identifiers: resolve to a person (email, phone, names, addresses)
- Identity management is built around keys like (cookies, hashed emails, IP addresses, and customer IDs)
- Identity management is about matching one to the other – they can be probabilistic or deterministic
4) Segmentation
- The practice of grouping customers or accounts together based on similar characteristics
- Helps with data-driven approach to marketing. Segmentation can be used in many ways:
- Product development, pricing, targeting, messaging and measurements.
- Smart segmentation is a key step toward meeting customer’s demands for more relevant experiences.
- Different types of segmentation: Psychographic, Behavioural, Benefits, Geography, Occasions
- Can be identified manually or through clustering.
- Most common segments:
- Customer value – tiering by potential value to the company
- Product Preference – types of products bought
- Loyalty – share of wallet and stickiness, correlated to value but not the same as
- Price sensitivity – often tied to product margin (business vs personal buyer)
- Use – Depth of engagement or frequency of use
- Perception that 5-8 segments is best – but you can have as many as are useful
- Attributes are really important to segmentation. Data harmonization and identity management are requisites
- Attributes come in three basic types (are, do, think)
Type | Uses | Data Type | Sources |
---|---|---|---|
Are | – Media targeting – High-level filters – Offline media buying – Predictors for attitudes | – Demographic – Filmographic – Income + credit – Family size | – CRM systems – Marketing Service Providers – Public sources – Third-party sources |
Do | – Product Preference – Channel preference – Locations visited – Price sensitivity – Content interests – Likelihood to respond | – Online behavior – Purchases – Location data – Devices used – Social media use – Content consumption | – Campaign management systems – Point of sale – Digital analytics – Email services – Media platforms |
Think | – Messages – Advertising Campaigns – Competitive positioning – Call scripts | – Psychographic – Affiliations – Sentiment and valence – Responses to content | – Inference models – Surveys – Social analytics – Voice of customer |
- Businesses love to be able to explore customer profiles with queries → to help with hypothesis and experimentation.
5) Activation
- Taking all the collected, identified, harmonized, and segmented customer data and putting it to work → to drive operational systems
- Activation intersects with engagement channel technology
- Campaign management tools, email, mobile and social platforms
- The other systems execute decisions developed, managed, and tracked based on the data and profiles sitting within the customer data platform
- Key Activation Activities (how the platform communicates with engagement systems):
Engagement Activation | – send segments to engagement systems – personalization of websites, dynamic creative – sequenced multi-step journeys – A/B testing support |
Realtime Event Based Marketing | – triggered messages and other treatments – predictive sending and scoring |
Predicative Analysis | – product recommendations – content recommendations |
Reporting and Insights | – dashboard / reporting – AI consumer insights |
- The platform needs to be able to send segments to many different channels
- Realtime event-based marketing requires fast response times (e.g. product recommendations)
- Should support File transfer, APIs and Queries.
Chapter 5 – Build a first party data asset with consent
- Consent and compliance are part of a transparent, trusted data-value exchange with customers
- Customers are increasingly resistant to data collection
- Customers are increasingly resistant to advertising
- 4 parties involved in data privacy: Web Browsers + standards bodies, government regulators, consumers and companies
- Browser Market Share: Chrome 65%, Safari 18%, Edge 4%
- Safari blocks third-party tracking cookies by default
- Google ending third-party cookies by end of 2023
- The future is first-party data, consumer who opt in and the use of aggregate rather than user-level information
- The Seven Principles of GDPR
- Transparency → the consumer should know what data is being collected by whom
- Purpose limitation → data can only be used for the explicit purpose granted by the customer
- Data minimization → only as much (and no more) data can be collected to serve the purpose above
- Accuracy
- Storage limitation → limits to how long data can be retained
- Security → must be stored in a way that it cannot be accessed by unauthorized parties
- Accountability → the data ‘controller’ must provide ability to audit and edit data
- Marketers must collect consent alongside data, and provision the infrastructure to be compliant with GDPR
- Practical level you need to classify data, determine user entitlements and ensure compliance
- 6 ways to build trust
- Get consent
- Restrict processing of data
- Honor the right to be forgotten
- Allow subject requests for data stored
- Keep data secure
- Capture data use preferences
- 4 ways customers feel
- Lack of control 81%
- No benefit 81%
- Confusion 59%
- Paranoia 72%
- Customers are happy to share if you’re transparent, there’s a clear value exchange and you give the consumer control
- Consumers will happily share data with companies that they trust
- 4 Ways to gain trust:
- Reliability: security and uptime
- Legality: compliance and flexibility to react to legislation
- Transparency: collection and use of customer data
- Value: sufficient benefits received for exchange
- A healthy majority of customers are willing to share data in return for relevance – as long as it is collected and used in a way that makes them feel comfortable
- The Privacy Paradox:
- We all say that we value our personal data, but we regularly surrender intimate information to platforms such as Google, Facebook, Amazon etc.
- Prices for adverts on Safari are 50-60% lower because of the banning of 3rd party cookies
Chapter 6 – Building a Customer-Driven Marketing Machine
Know, Personalize, Engage and Measure
Know your customer as much as you can through data collection
- Capture data across the known and unknown modalities
- Capture all of information customers decide to reveal (email, preferences)
- Capture what you can infer about their intent (clicks, video views)
- Build the source of truth
- The amount of data that’s consented to is important
- Garbage in garbage out
- You need to build the right dataset – requires consent, truth (resolve identity) and scale (to power AI)
Personalize every interaction (the right message)
- Email is like an ATM machine – but people are getting too many emails as a result
- If personalization can help improve each step of the funnel – then the effects on the total funnel are much larger (10% each step might add up to 50% for the campaign)
- Need to personalize through the entire engagement layer
Engage across the channels and surfaces they touch
- Marketing to e-commerce, to call center, to real life experiences
- Get close to your loyal customers
- Make sure each touch-point is aligned and operates in close to real time
- Connecting the touch points can increase lifetime value
- Start with connecting advertising to e-commerce (stop showing them things they’ve bought and start showing them things they might buy)
- Journey Management
- Manage them in a prescriptive way
- Provision them in real-time – as the engagement is happening. Real-time interaction management (RTIM) requires that different tools all have real-time capability
- can help you connect and relate fast-moving experiences, driving relevance and delivering lift
- Orchestrate the way your customer engages across a number of channels
- Journey management helps wring maximum value out of known customers through personalization
Measure everything and use that data to improve
- Analytics, measurement and attribution
- Knowing, personalizing and engaging leads to insights about your customer
- Liberate measurements from silos so they can inform each other
- Optimize the experience and value from paid marketing
Organizational transformation
Goal: get better at understanding and engaging with their customers
The software itself cannot solve the problem alone
Transformations come together around people, process and technology
While you need all three, the people are probably the most important aspect
Conway’s Law: Any organization that designs a system will produce a design whose structure is a copy of the organizations communication structure.
Conway’s law describes the process by which companies operate based on the structure of their org chart
In every successful implementation we have witnessed, at least one senior executive from these departments has worked to form a center of excellence approach that made data management a top company priority
You need a platform operating philosophy. A common language, enables creativity by allowing extensible model that lets people develop on them:
- Platform approach is what gets value from the CDP
- Democratize data in the organization. Make data easily available to anyone who needs it
Data unification for it’s own sake doesn’t provide value
Broad and aspirational CDP use cases are needed to start the project:
- Get the company aligned about near term success
- Showing the exec the juice is worth the squeeze
- Every use case needs refinement so that it drives a specific outcome.
- E.g: Reduce the cost of customer acquisition → refine customer segmentation to increase conversion rates on targeted campaigns.
- E.g: Know more about my customer → Combine POS with digital display data to better understand video media in key segments to increase conversion rates by product
- You can’t track the effectiveness of your data transformation without a clear performance framework – and having KPIs that align with is paramount. Many companies start with simple KPIs that show platform adoption
Methodology: supporting a culture that allows for inquiry and allows for fast results through testing and rewards data-driven insight.
Operating Model
- Fund ongoing executive support by aligning data transformation goals with the CEO and CFO
- CDP should be Capex – the CDP is a core piece of tech that no company can live without – the system of record for customer data
- What is the entry point that gets exec support?
- Cost savings, security or operations. A combination of all three will get you funding
The Center of Excellence Model
- What about the people stack required to achieve success?
- People, process and technology.
- Marketing, technology and Analytics stakeholders are key
- Marketing must represent the need for customer data as the unifying thread that connects advertising, messaging and website and app experiences across the organization. The CMO must own the CDP.
- Technology – CDP must be the single truth for more that just marketing data. Bless the CDP for capturing, storing and unifying customer data. Migrating things over, owning the process.
- Go from being data gatekeepers to data retailers
- In exchange for giving everyone access → everyone must enrich the CDP data by returning relevant data → stream attribute data back to to the data store
- Analytics → own the segmentation strategy, and set the metrics for success by building models that can measure the value that customer data brings to the organization
- create rich and dynamic segmentation
- should also help create the ROI model – establish KPIs
- The idea is to produce a flywheel
- Tech gives access to highly enriched and unified customer data set
- Analytics team builds better customer segments
- Marketing activates segments
- Campaigns create more data that further enrich the dataset
- Better data leads to better segmentation. Better segmentation leads to better engagement. Better engagement leads to better data. Rinse and repeat
A working maturity model
- Channel Coordination (single, multi, cross, omni)
- Engagement Maturity (segmented, lifecycle, individualized, connected)
- Segmented → by segment
- Lifecycle → by segment + funnel stage
- Individualized → customer + funnel stage + status
- Connected → profile + different systems + personalization
- From touch-points → journeys → experiences
- Journeys requires multi-channel and better identity capability and better lifecycle awareness
- Experience → move from channel based interactions to real time in the moment sending the right message and creative
Chapter 7 – Adtech and Data Management Platform
- AdTech data is often double siloed – consigned to agency partners without much data sharing
- DMPs helped capture, unify and activate through cookies – caused a 10x price increase in ad price for the right customers
- Publishers first used the DMPs – deep segmentation yielded more value from advertisers
- A typical user had 20 cookies, multiple mobile ad IDs, and interacts with a number of connected devices
- This is pseudonymous identity data but it can still make a rich profile
- 5 Sources of value in DMP
- Audience planning
- Audience data activation
- Personalization
- Campaign optimization
- Delivering insights
- DMPs were the single source of truth of people when they appeared in a pseudonymous state
- Any brand suffers form observational bias – if you only understand your customers and not the market
- You need to go beyond your own customers data to find and acquire new customers and fill the top of the funnel and drive new revenue
- Those customers are on social networks, they’re browsing your site anonymously
- How do you reach them? Through advertising – How do you target them? Using the DMP and building segments of pseudonymous consumers
- Why Pseudonymous data is still important
- The number of internet connected devices is only going to increase
- Most consumers interact with brands anonymously before they volunteer information
- Consumers digital exhaust signals can reveal a deep understanding of intent
- Capture, unify and activate pseudonymous data to connect the customer journey, enrich the knowledge of your customers, and reach net-new customers at the top of the funnel
- Ad networks let you send first party data to them – so you can target the customers you know (by email address) etc. Facebook will return a match about 70% of the time.
- CDPs therefore can define a segment and send it to Facebook or google and execute an ad campaign against it
- CDPs and DMPs are on a crash course to become CDMPs
- The best of DMPs (pseudonymous data capture, consent management, real-time profiles, cross-device identity management) functionality will be required in CDPs
- Creating a single source of truth for known and unknown, and consent, and segmentation
Chapter 8 – Beyond Marketing
- Customer data can make a better experience across marketing, support and sales
- Customers data must be at the center of all our systems – the person should be the currency of identity
- An experience can either be seamless or disjointed, depending on how good companies are at using customer data
- Abandoned baskets are notices and customers are emailed.
- Purchases are noticed and customers are emailed (new purchase flow)
- A more seamless experience can increase the LTV of customers and reduce churn
- What if the call center knew your persona / priorities (prioritize location, price, outcomes etc) and was able to tailor their reaction to you
- Persona-based contextual buyer consideration journey – connecting service and marketing
- Start with suppressing adverts to customers who’ve already purchased that product to reduce costs
- Once a customer has purchased we have high value deterministic data to develop propensity models
- Connect this data to unknown users. You could send emails for abandoned baskets
- Combining highly granular attributes of unknown visitors with known customer purchase data can yield effective predictive models
- The purchase isn’t the end of the journey → but the seed that can grow into a new top-of-funnel campaign
- The single source of truth → creates a platform that others can build on
- House-holding → target households not just individuals
- Track targetable attributes → engagement of emails can help with propensity modelling
- How can marketing data be an input into the sales, service and commerce functions
- Every team gets more in value than they individually contribute (Platform / Center of excellence mindset)
Chapter 9 – Machine Learning and AI
- AI started in the 1950s – attempt to make computers do things humans do
- Weak AI – specific problems (like interpreting language)
- Strong AI – build more general systems
- Model that exports a label (Hotdog or Notdog)
- Customer data in a warehouse serves no purpose – it must be activated to yield value
- Two types of activation
- Operational Activation: data is put to use in a process like message personalization
- Analytical Activation: ML and AI
- Marketing Data Scientist Tasks:
- Measurement, optimization, experiments, segmentation, predictive modelling, story telling
- Quantitative skills + knowledge of the industry + curiosity
- Data Science domains
- Exploration: using statistics and visualization techniques to find patterns in data
- Experimentation: applying design of experiments methods to develop and test hypotheses under controlled conditions
- ML and AI: applying algorithms to build models and make predictions
- Spend most of their time locating and cleaning data, or feature engineering → working out what attributes are more meaningful
- Defining problems, selecting and exploring data, trying different solutions and weighing trade-offs among them – not usually, writing algorithms
- Common marketing data experiments
- different version of a website or content
- different email subject lines and mobile push messages
- item recommendations or incentive offers for different groups
- test impact of create treatments or media placements for advertising
- ML and AI
- About making predictions or finding underlying structures in data
- What is a model?
- Training – fitting a model to data
- Testing – validating the effectiveness of the fitted model
- Labelled data is data for which the desired outcome is known, and it is required to build predictive models
- Clustering can be used to identify different groups or determine some other underlying structure in the data
- No model is perfect – some are more useful than others. The analyst must balance between the two extremes of
- Memorizing the training (or overfitting)
- Building a weak model (or underfitting)
- Supervised vs Unsupervised
- supervised – using labeled data to build models that make predictions
- unsupervised – applying methods to unlabelled data to identify structures
- Most marketing prediction models are supervised and use labeled historical data to build a model that can make predictions
- Regression = Predicting a numerical value
- Use numerical data to make a prediction of a desired value
- Estimating LTV of a new customer based on their first 90 days of purchases
- Predicting incremental orders following an advertising campaign
- Determining the best frequency to send emails based on historic open rates
- Use numerical data to make a prediction of a desired value
- Classification = Classifying customers and prospects
- Rather than predict a numerical value, categorical models attempt to classify or label something
- Predict whether someone will or will not click on an ad
- Determine whether someone is likely or unlikely to purchase a given product
- Identify B2B sales leads who are more likely to respond to a pitch
- Rather than predict a numerical value, categorical models attempt to classify or label something
- Clustering: Finding structure in numerical data generally involves creating groups or clusters that have some internal similarity while being relatively distinct from other groups
- Discover different groups of visitors to a marketing website based on browsing behavior
- Determine different types of prospects based on demographics and buying patterns
- Separate high-value customers into subgroups based on preferred product types
- ML can help find structure that is not obvious to an unaided analyst
- Dimensionality Reduction
- A variety of complex methods are used to detect underlying structure within categorical datasets (or combined numerical datasets)
- Determine which items are to recommend to a customer based on his similarity to other customers
- Find evidence of brand affinity on social networks based on identifying logos in photos
- Divide customer comments into different topics based on linguistic analysis
- A variety of complex methods are used to detect underlying structure within categorical datasets (or combined numerical datasets)
- Neural nets
- Deep learning models. Training a network of nodes in various ways, to mimic functions of the human brain. More suited to address highly nuanced and data-intensive tasks such as image recognition (where is the cat in the picture?)
- Top uses for AI among marketers
- Personalize individual channel experiences 80%
- Improve customer segmentation / lookalike audience modelling 77%
- Automate interactions over social channels / messaging apps 76%
- Drive next best actions in real time 76%
- Surface insights from data 78%
- Machine Learning segmentation
- Much of segmentation is still trial and error (despite realtime reporting and A/B testing)
- Machine-Learned segmentation can utilize rich data from historical campaigns and determine better segments for future campaigns by analyzing which attributes really make people buy
- Machine-Learned attribution
- There are ways to measure and attribute success to a multichannel journey
- Accuracy depends on the values you assign to different interactions
- Last touch attribution – values the last interaction {most commonly used}
- Linear attribution
- Time discounting
- Sequence discounting
- ML can look across thousands of journeys and assign values to various touchpoints
- Can look at the most effective sequence
- Image recognition and Natural language processing
- Image recognition use cases:
- Social: recognizing brand logos in social posts
- E-Commerce: automate previously manual tasks (labeling, classification of inventory)
- Recommendations: recommendation algorithms
- Personalization: automating testing and learning process to include
- Search and visual search
- NLP use cases in marketing:
- Social and email: text editing, AI recommendations to improve emails,
- Customer experience: Summaries and sentiment analysis
- Content creation: NLP autogenerate text (subject lines etc)
- Chat-bots: service applications, to cross-sell, up-sell etc
- Voice applications: create voice communications
- Image recognition use cases:
- Can marketers make use of the talent sitting inside and outside company walls?
- Don’t assume all data scientists are equal
- Provide opportunities for data scientists to learn on the job.
- Understand the basic domains of data science
Chapter 10 – Orchestrating a Personalized Customer Journey
- Marketing Saying: Right person, Right message, Right time.
- Likeliness to buy = mental availability * physical availability
- Brands can demand a premium if they align to people’s identity and trigger positive thoughts
- Brands need consistent messaging, and to be readily available where the customers are buying
- In 1978 Gary Thuerk sent the first marketing email on Arpanet to 400 people promoting DEC computers → it created $13M in sales
- By 2010, segmenting email lists and using contextual and behavioral attributes to microtarget customers was more common.
- 2010 started automating campaigns ‘Customer Journey Management’ is the ability to provision a series of interactions based on a customer’s stage in a process
- Customers behave similarly → its efficient and effective to automate common journeys
- Most have mastered shipping updates → harder to unify customer service etc
Predictive Journeys
- Delivering a better journey based on prediction is ‘true journey based marketing’
- Email campaign Example:
- Customer on web utilizes promotion, puts an item in the cart but walks away. It could be because
- got distracted or didn’t have time to complete
- had reservations about the cost (or shipping costs) and abandoned their cart
- left to find a better deal elsewhere
- You could create a different journeys to address these issues
- Customer on web utilizes promotion, puts an item in the cart but walks away. It could be because
- Intelligent journeys use rules-based methodology to send customers down a path that advances through time / or steps
- Persona Split → build journeys based on contact-level data
- Engagement split → segment journeys based on channel we predict consumers are likely to engage on
- Call centre, ad, email etc
- AI can use predictions to create journeys across multiple channels that increase conversion rates, reduce churn, and join touchpoints like service and commerce
- The more data you have – the smarter your decision tree can be:
- Marketing, Commerce, Service, LTV, Propensity to buy
Real-Time Interaction Management
- Delivering iterative contextually relevant experiences, value, and utility at the appropriate moment in the customer lifecycle via preferred customer touch points
- Most journey management tools are email based, which doesn’t cover unknown customers
- RTIM tools can make immediate personalization decisions
- It’s challenging as journeys are often multi-day, multi-channel and multi-device
- CDPs make this easier
- Speed, intelligence and channel coordination
- RTIMs deliver the ‘Next Best Action’ or ‘Next Best Offer’ at the right moment across channels
- even deployed simply – you can lift conversion rates by bringing users back to the last page they visited
- Having the capability to build predictive journeys – and to manage interactions in real time across channels
Chapter 11 – Connected Data for Marketing Analytics
- Value Chain of Business Intelligence
- Business Understanding → ← Data Understanding
- Data Understanding → Data Preparation → Data Modelling → Evaluation → Deployment
- Business Understanding → ← Data Understanding
- The Marketing Department and CMO are responsible for
- Understanding markets and customers
- Create strategy and marketing assets
- Manage all the functions required to execute marketing and programs and operations
- Some of the most critical categories of support include:
- segmentation
- customer lifetime value
- campaign and channel measurement
- media mix and attribution
- predictive and propensity modelling
- social analytics
- dash-boarding and visualization
- For analytics – additional data sources might include
- 3rd party data and overlaps
- voice-of-customer (inc. call center output)
- product and pricing data
- loyalty systems
- trade promotion and event analytics solutions
- Advanced Marketing Analysis
- Multi-channel
- Nontraditional data → location, social, text and speech analysis, voice of customer, IoT
- Greater scale → more that just SQL
- Realtime analysis → event processing, analysis of data in motion
- Predictive models → next best offer and content recommendations, brand and churn propensity, profitability and lifetime value
- More data types → what people are (demographics), what they do (behaviors) and what they think and feel (psychographic and attitudinal)
- Email Analytics
- Layer One: Open rate, click through rate and opt-outs or unsubscribes
- list growth, deliverability and unsubscribe rates
- Layer Two: Demographics, look at performance through age, gender and location impact campaign performance
- Layer Three: A/B testing: how components impact engagement: headline, offer type, product etc
- or different times of day for different groups
- Layer One: Open rate, click through rate and opt-outs or unsubscribes
- Data Management Platform Analytics
- Slice analytics by vendor and segment
- How did this campaign perform for a particular group (male versus female) or a vendor (Yahoo versus Google)?
- Which audiences converted faster, based on their exposure to advertising, and manage the delivery of cross-channel advertising
- The “sweet spot” in monthly ad delivery. People tended to click, buy, or “convert” when seeing between 5–20 ad impressions per month.
- Less is ineffective (people need multiple impressions to enter into brand consideration)
- More than that is wasting money – banner blindness
- DMP people based view changed the game. A CDP that unifies people data from both pseudonymous and PII-based sources widens the aperture of analytics by introducing attributes that common media analytics never really considered, such as historical purchase data, call center interactions, and loyalty data.
- Slice analytics by vendor and segment
- Multitouch Attribution (MTA)
- Consider the impact of one or more customer interactions that lead to a KPI (a sale, a download, a click)
- Tend to be digital-only in scope due to data
- Traditional attribution models bluntly attribute to a single touch point, for example…
- first interaction (search result)
- last interaction (used a promo code)
- Those models are ruined by the fact that many journeys start and end with a Google Search
- MTA attribution modes assign value to the interactions across the journey (emails, website visits, video views, in-store visits). For example…
- equal value to every touchpoint
- equal value with time decay (closer to the sale is more valuable)
- Media Mix Modelling (MMM)
- MTA’s but aggregated
- Built at campaign level, best bet for attributing offline (TV, print, terrestrial radio) media with digital
- Can look beyond the immediate time period, and consider several years’ worth of media spend
- 10,000-foot view of how promotions or seasonality affected sales
- CPGs and retailer typically have built robust MMM practices over time, and found them reliable sources of directional attribution for hard-to-measure offline sales, and barometers of soft metrics like brand equity
- In future we’re going to see less user-level data released → working with aggregate data is going to be more common → moving measurement away from log files and user-level analytics such as MTA
- Marketing Analytics Platforms
- Manual / Batch Dashboards
- Crafted marketing performance dashboards and exposed key reports to inform periodic optimisations.
- track a campaigns KPIs over it’s lifetime – set alerts for high/low performance
- Used batch data and careful manual work.
- As a result updates were infrequent, as were optimisations based on the insights shown
- AI has helped with:
- data capture (smartly fitting disparate data sets together in a persistent model),
- analysis (deep learning to surface anomalies in the data)
- and activation (automated optimization recommendations)
- AI / ML has transformed dashboards into intelligence tools that increase time to value by automating the process of data unification, building data models, and making optimisation available hourly (rather than weekly or monthly)
- The CDP unifies people data – The right marketing analytics dashboard unifies cross-channel interaction data
- A powerful combination
- Manual / Batch Dashboards
Chapter 12 – Summary and Looking Ahead
- Start sales presentations with a “forward-looking statement”
- But customers should make investment decisions based on what we have available to offer today (not our future roadmap)
- The move toward aggregate level data is not going away
- Shift from user-level data analytics to aggregate-level data
- Walled-garden data environments like Google, Facebook, Amazon, Apple
- + continuing privacy legislation by governments
- + properly collected and consented-into first-party data is going to be more valuable than ever
- CDPs should have powerful data provisioning services (for replacing third-party marketplace data with second-party partner data)
- for clean-room data sharing to suck insights out of walled gardens
- MTA and other measurement disciplines are too dependent on digital marketing touchpoints (clicks, email opens, video views, etc.), and biased to data points that are easily captured in systems
- We’re going to have to make the most of what we have (sales, service and commerce)
- Shift from user-level data analytics to aggregate-level data