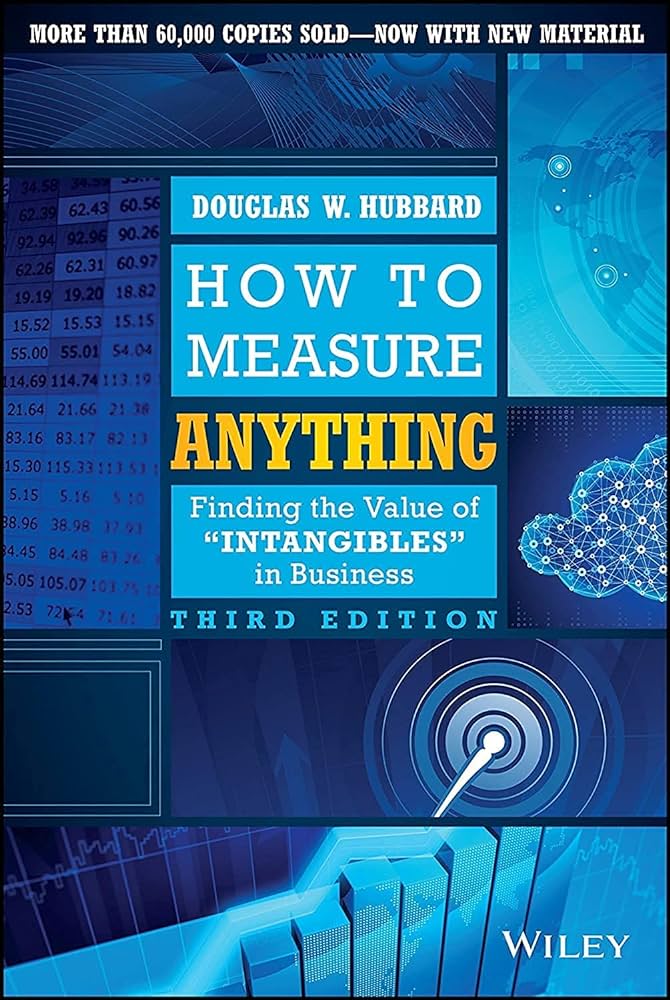
Review
“How to Measure Anything” by Douglas W. Hubbard is a must-read for product managers, despite not being directly related to product development. This book provides invaluable insights into the art and science of measurement, which is crucial for successful product discovery.
As product managers, we often face uncertainty when exploring new ideas and validating assumptions. Hubbard emphasizes that measurement is about reducing uncertainty, and even small amounts of early observations can be incredibly informative in the face of uncertainty. This concept is particularly relevant to product discovery, where we continuously seek to learn and make informed decisions based on data.
Throughout the book, Hubbard presents a framework for quantifying seemingly immeasurable things, such as user preferences, market demand, and the potential impact of new features. By applying these techniques, product managers can make more accurate predictions, prioritize effectively, and allocate resources wisely.
One of the key takeaways from this book is that measurement doesn’t always require precise data or extensive analysis. Often, rough estimates and simple observations can provide valuable insights and help guide decision-making. This understanding can empower product managers to take action even when faced with limited information.
While “How to Measure Anything” is not a quick read, it is well worth the investment.
Key Takeaways
The 20% that gave me 80% of the value.
- Anything can be measured. If it matters at all, it can be observed and measured.
- The concept of measurement as “uncertainty reduction” and not necessarily the elimination of uncertainty is a central theme of the book.
- Measure what matters to make better decisions. Focus on high-value measurements.
- Define the decision.
- Determine what you know now.
- Compute the value of additional information. (If none, go to step 5.)
- Measure where information value is high. (Return to steps 2 and 3 until further measurement is not needed.)
- Make a decision and act on it (Return to step 1 and repeat as each action creates new decisions).
- Definition of Measurement (an information theory version): A quantitatively expressed reduction of uncertainty based on one or more observations.
- This “uncertainty reduction” is critical. Major decisions made under a state of uncertainty and when that uncertainty is about big, risky decisions, then uncertainty reduction has a lot of value—and that is why we will use this definition of measurement.
- The Baysian Interpretation of Measurement → when probability refers to the personal state of uncertainty of an observer or what some have called a “degree of belief.” Bayes’ theorem describes how new information can update prior probabilities. The prior probability often needs to be subjective
- Break down complex problems into estimable parts. Decomposition alone can significantly reduce uncertainty. It gives the estimator a basis for seeing where uncertainty about the quantity came from.
- You often have more data than you think. Look for creative ways to gather relevant information.
- Statistics courses should teach that even small samples can improve decision-making odds when uncertainty is high.
- Most measurements people regard as difficult involve indirect deductions and inferences. When you need to infer something “unseen” from something “seen.” Eratosthenes couldn’t directly see the curvature of Earth, but he could deduce it from shadows and the knowledge that Earth was roughly spherical.
- Inference examples:
- Measuring with very small random samples of a very large population
- Measuring the size of a mostly unseen population
- Measuring when many other, even unknown, variables are involved
- Measuring the risk of rare events through observation and reason.
- Measuring subjective preferences and values: by assessing how much people pay for these things with their money and time
- The Rule of Five: There is a 93.75% chance that the median of a population is between the smallest and largest values in any random sample of five from that population (regardless of the population size). The range will be wide but it’s narrower than having no idea.
- The Single Sample Majority Rule (i.e. The Urn of Mystery Rule) Given maximum uncertainty about a population proportion, such that you believe the proportion could be anything between 0% and 100% with all values being equally likely, there is a 75% chance that a single randomly selected sample is from the majority of the population.
- Usually only a few things matter—most of the variables have an “information value” at or near zero. BUT some variables have an information value that is so high that some deliberate measurement effort is easily justified.
- There are variables that don’t justify measurement, but it’s a misconception that unless measurement meets an arbitrary standard (statistical significance) it has no value.
- A decision has to be defined well enough to be modeled quantitatively.
- What makes a measurement of high value is a lot of uncertainty combined with a high cost of being wrong.
- Don’t assume only direct answers to questions are useful. Indirect observations can be valuable.
- Calibration training can improve intuition for assigning probabilities and ranges to uncertain quantities. Better estimates are attainable when estimators have been trained to remove their personal estimating biases. Putting odds on uncertain things is a learnable skill. For range questions you know of some bounds beyond which the answer would seem absurd.
- Tips to help improve your confidence interval intuition:
- To calibrate better pretend to have an equivalent bet with a 90% payoff, move your confidence interval bounds until you’re indifferent between the two.
- Repetition and feedback: It takes several tests in succession, assessing how well you did after each one and attempting to improve your performance in the next one.
- Consider potential problems: Look to identify potential problems for each of your estimates. Assume your answer is wrong and try to explain to yourself why.
- Avoid anchoring: Look at each bound on the range as a separate “binary” question. For a 90% CI you must be 95% sure that the true value is less than the upper bound. Increase the upper bound until you’re that certain.
- Reverse the anchoring effect: Instead of starting with a point estimate, start with an absurdly wide range and then start eliminating the values you know to be extremely unlikely. What values do I know to be ridiculous?”
- Tips to help improve your confidence interval intuition:
- Risk is a state of uncertainty with potential negative outcomes. Quantify risks with probabilities and impacts.
- Using all optimistic values for the optimistic case and all pessimistic values for the pessimistic case is a common error and no doubt has resulted in a large number of misinformed decisions. The more variables you include, the greater the exaggeration of the range becomes.
- Monte Carlo simulations can model risks by generating scenarios based on input probabilities.
- Expected Opportunity Loss (EOL) is the chance of being wrong times the cost of being wrong.
- The Expected Value of Information (EVI) is the reduction in EOL from a measurement. It guides measurement efforts. The point of measurement is to reduce the uncertainty, you therefore reduce expected opportunity loss.
- A common misconception is that massive data is needed to gain useful insight when uncertainty is high. In reality, is you have high uncertainty, a small amount of data can significantly reduce uncertainty.
- If you’ve never measured it before, you may lack even a fundamental sense of scale for the quantity. So, the things you measured the most in the past have less uncertainty, and therefore less information value, when you need to estimate them for future decisions.
- Measurements have a time-value constraint therefore prefer small, iterative observations.
- The Economic Value of Information is often inversely proportional to the amount of measurement attention a variable gets (Measurement Inversion).
- You almost always have to look at something other than what you have been looking at in the past.
- The Risk Paradox: If an organization uses quantitative risk analysis at all, it is usually for routine operational decisions. The largest, most risky decisions get the least amount of proper risk analysis.
- Instruments of measurement, while imperfect, offer advantages over unaided human judgment alone.
- Decomposition
- Decomposition involves computing an uncertain variable from less uncertain or more easily measurable components.
- Decomposing a variable into observable parts can make measurement easier.
- Decomposition alone often sufficiently reduces uncertainty, requiring no further observation (decomposition effect).
- Nearly 1/3 of decomposed variables need no additional measurement.
- The decomposition process itself reveals that seemingly immeasurable things can be measured.
- Following the trail: Observation:
- Follow its trail like a clever detective. Do forensic analysis of data you already have.
- Use direct observation. Start looking, counting, and/or sampling if possible.
- If it hasn’t left any trail so far, add a “tracer” to it so it starts leaving a trail.
- If you can’t follow a trail at all, create the conditions to observe it (an experiment).
- The information value curve is usually steepest at the beginning. The first 10 samples tell you a lot more than the next 10. The initial state of uncertainty tells you a lot about how to measure it.
- Consider potential errors and biases in measurements, but don’t be paralyzed by them. Some measurement is better than none.
- Controlled experiments compare test and control groups to establish causation, not just correlation.
- Absence of evidence is evidence of absence, contrary to the common saying.
- Surveys should avoid response bias through careful question design and behavioral observation.
- The value of additional measurement drops off quickly. Focus on initial high-value measurements and reassess.
- A measurement framework: Define decisions, model uncertainty, compute information value, measure, decide, repeat.
- People tend to measure what’s easy, not what’s important. Focus on high-information value variables.
- Managers often prefer measuring factors that produce good news. Avoid this bias.
- If you’ve never measured something before, you likely lack a fundamental sense of scale for it.
- Not knowing the business value of measurements leads to over-measuring low-value factors.
- Be resourceful and clever in collecting relevant data. Squeeze more out of limited information.
- Iteratively measure in small steps. Initial measurements often change the value of further measurement.
- Challenge assumptions about immeasurable factors. Measuring the seemingly immeasurable is often quite feasible.